PPM-CNN
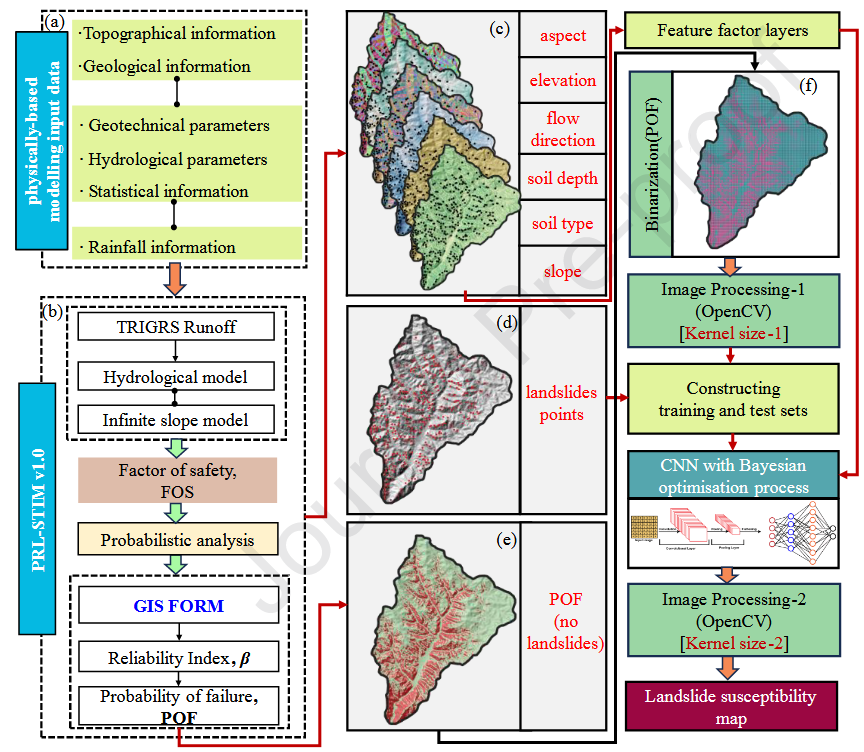
Landslide susceptibility mapping (LSM) plays a crucial role in assessing geological risks. The current LSM techniques face a significant challenge in achieving accurate results due to uncertainties associated with regional-scale geotechnical parameters. To explore rainfall-induced LSM, the study proposes a hybrid model that combines the physically-based probabilistic model (PPM) with convolutional neural network (CNN). The PPM model is capable of effectively capturing the spatial distribution of landslides by incorporating the probability of failure (POF) considering the slope stability mechanism under rainfall conditions. This significantly characterizes the variation of POF caused by parameter uncertainties. CNN is used as a binary classifier to capture the spatial and channel correlation between landslide conditioning factors and the probability of landslide occurrence. OpenCV image enhancement technique is utilized to extract non-landslide points based on the POF of landslides. The proposed model considers physical mechanics comprehensively when selecting non-landslide samples, which can effectively filter out samples that do not adhere to physical principles and reduce the risk of overfitting. The results indicate that the proposed PPM-CNN hybrid model presents a higher prediction accuracy, with an area under the curve (AUC) value of 0.85 based on the landslide case of the Niangniangba area of Gansu Province, China compared with the individual CNN model (AUC = 0.61) and the PPM model (AUC = 0.74). This model can also consider the statistical correlation and non-normal probability distributions of model parameters. These results offer valuable insights for future research in conducting rainfall-induced landslide susceptibility assessment at the regional scale.